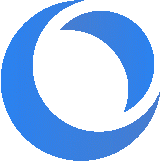
Objectives of the service
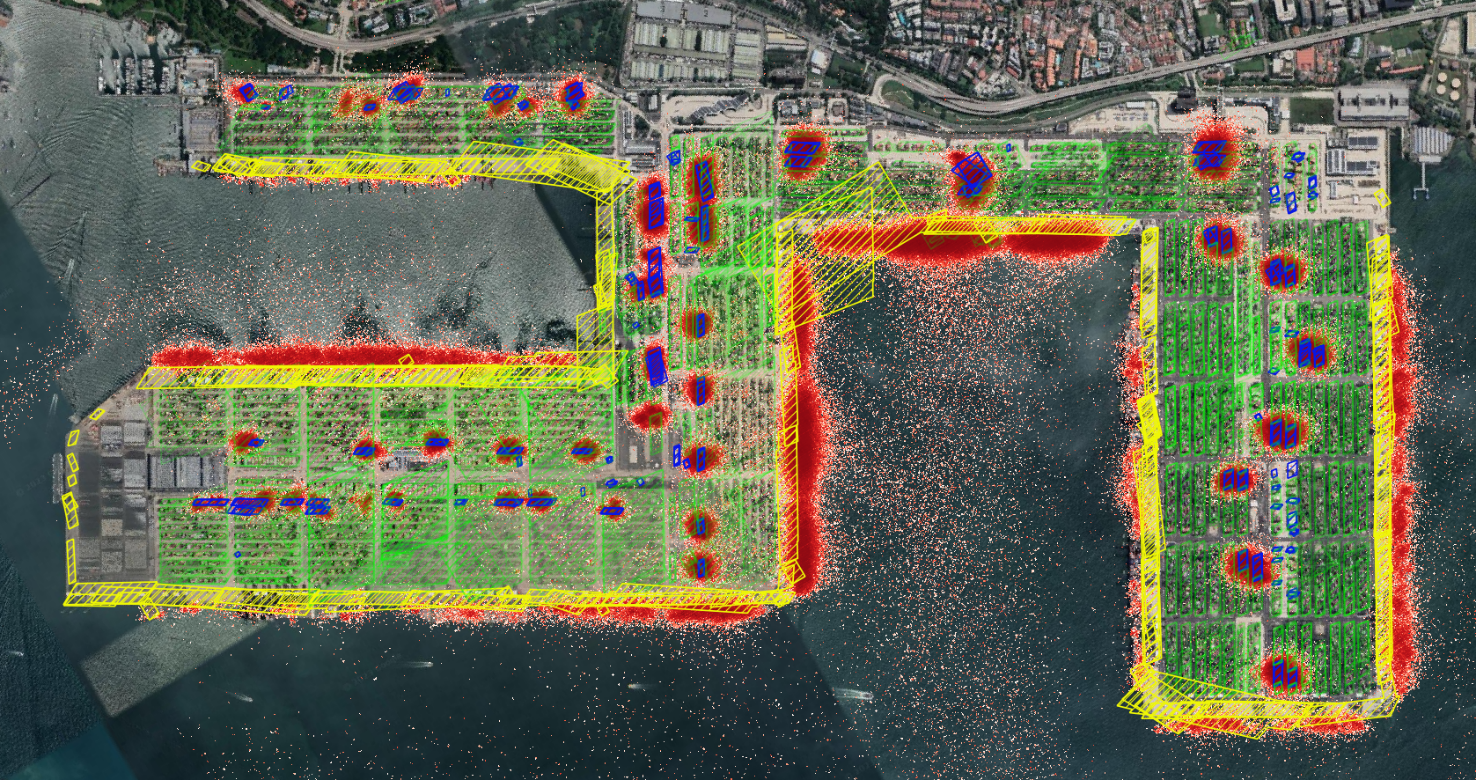
Bluepulse leverages AI to enhance supply chain operations and reduce cargo risks by tracking containers within port terminals. To do so, Bluepulse has developed the world's most extensive and precise container maritime terminal database from satellite images, enabling container tracking in all terminal areas.
Users and their needs
Shipping lines need increased container visibility and real time container tracking in terminal, where containers spend 1/3 of travel time on average. It also enables shipping lines and container operators to add context to container shipment and to qualify all container events (door opening, moves, loading), based on container exact locations.
The solution covers 1,041 maritime terminals in the world, covering 99% of intermodal shipping volume.
Service/ system concept
AI Deep Learning technology to detect 20 million containers in the world : VGG16 model made of 5 convolutional blocks to process efficiently large volumes of images and detect target images. | ![]()
|
Image classification algorithms to label each zone defined by their characteristics to tell apart each type of areas in terminals : OFFD, BRTH, COFS are DCSA terminologies for each activity of container handling in maritime terminals. | ![]()
|
Using API service, any container operator can query our database (GNSS coordinates) to retrieve exact container location in near real time.
The solution is deployed on a fault tolerant, horizontally scalable SaaS solution able to deliver 99.99% availability up time.
Results for these sample queries, showing Port code, Terminal code, Zone code and Zone type:
Here is a data query return from API (json file transformed into CSV):
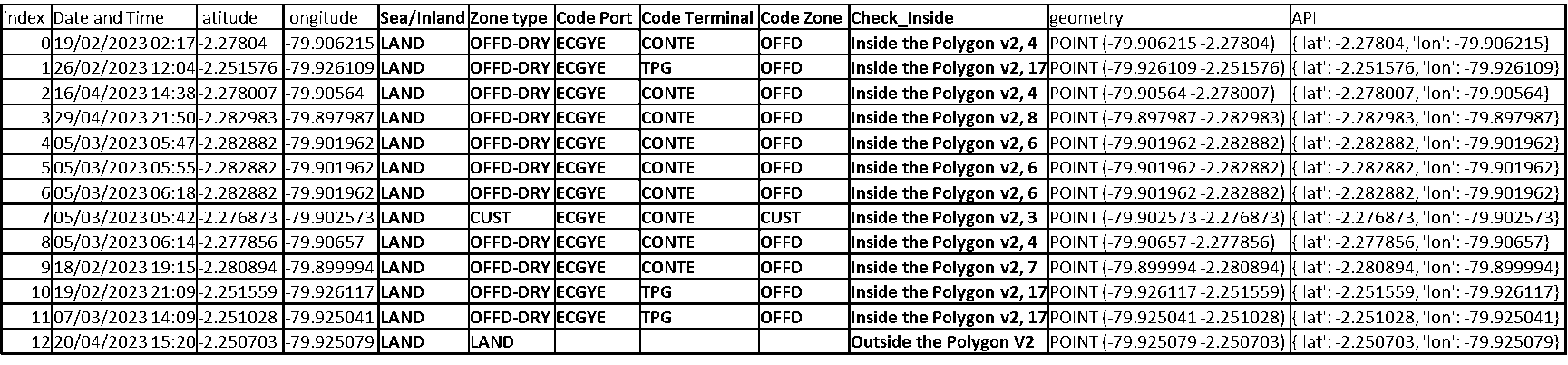
Space Added Value
Deep learning technologies is used to classify and segment ten thousand of high-resolution satellite images, covering over 100,000 km² of port areas in the world.
Several AI processes identify containers areas, and qualifies these areas to label their precise usage (storage, loading, quay, freight station).
Current Status
![]()
|
![]()
|
Over 1,000 terminal images have been collected, stored and processed; over 100,000 polygons created to represent all active container areas in maritime terminals.
Final user tests to measure solution accuracy using over 3,000 test data sets to compare solution results with field findings have been completed. Final review milestone of the activity took place in November 2023.